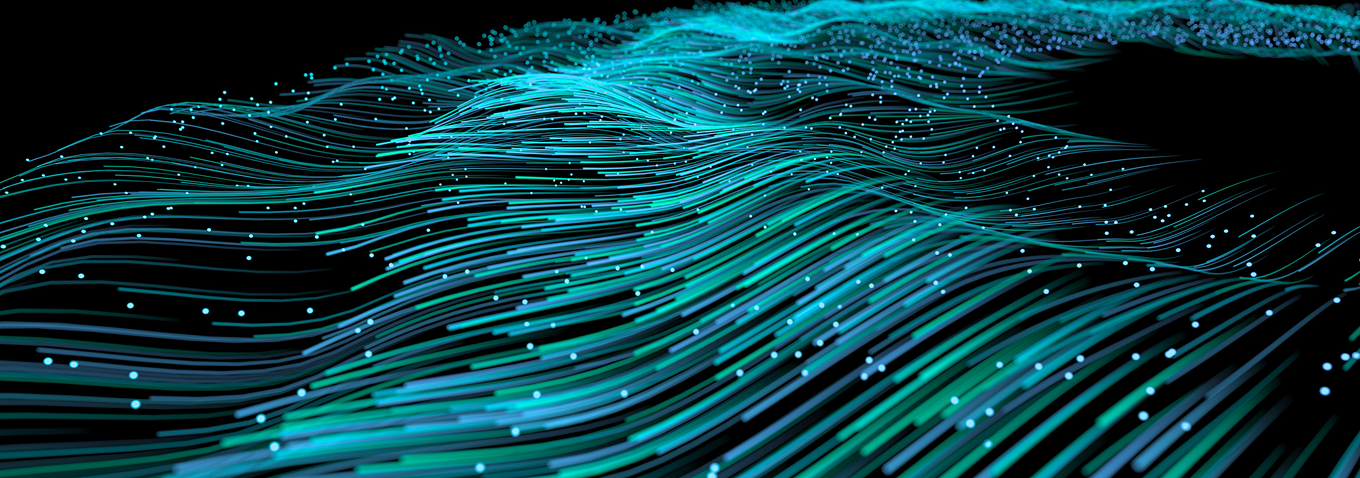
Data cleansing, also known as data cleaning or data scrubbing, is the process of identifying and correcting errors, inconsistencies and inaccuracies within a dataset.
It is a vital component of effective data management, helping to ensure that any given dataset within an organisation is accurate, consistent and reliable.
When an organisation’s data is flawed, inconsistent or incorrect, the insights which can be derived from it are likely to be unreliable and inaccurate. It can also waste time, result in ineffective decision-making, damage customer relationships and put a company’s reputation at risk. This is particularly true in financial services where even the smallest of errors can have huge impacts.
According to research and consulting firm Gartner, poor data quality costs organization an average of $12.9 million (around £9.9 million) every single year.
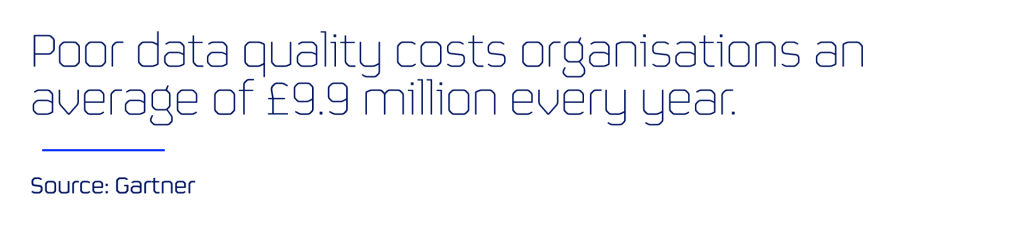
What are the benefits of data cleansing?
Improved data quality: by cleansing your data, you can reduce the chance of errors, inconsistencies or gaps. This helps improve the data’s reliability and means any outputs derived from its analysis are more accurate.
Better decision-making: consistent and accurate data allows organisations to conduct more comprehensive and in-depth analysis. Firms can then use the insights gained to make better, data-driven decisions and potentially uncover new revenue streams.
Increased efficiency: when data is inaccurate or incomplete, operational processes can become inefficient and error prone. By having access to clean and consistent data, firms can save time as errors will be addressed before they move further downstream.
Compliance: poorly maintained or inaccurate records can mean external data protection regulations or internal security policies aren’t well kept. This could lead to penalties and may even result in reputational damage for businesses. By keeping accurate and up-to-date data, and managing it well, firms ensure they remain compliant with necessary standards.
How do you cleanse data?
Organisations can clean their data manually or choose to automate the process using software tools. No matter which method a firm chooses, the data cleansing process will likely still involve several key tasks, each aimed at addressing specific issues within a dataset. Here are some of the most common tasks involved in the data cleaning process:
First, a business must identify and remove irrelevant or redundant observations from a dataset. This involves analysing data entries for duplicates, irrelevant information and errors. Anything irrelevant or redundant must then be removed.
Next, structural issues within the data must be addressed. This involves standardising formats to ensure uniformity. A common example of standardisation would be ensuring that dates are formatted in the same way within a dataset.
Businesses should then tackle any outliers or anomalies in their data. Sometimes, there are legitimate reasons a piece of data may not align with the rest of a dataset but it’s important for firms to assess whether this anomaly is legitimate or a mistake. If it is a mistake, then it should be removed to maintain the integrity of the data.
Finally, once these issues have been removed and formats have been made consistent, businesses should work to address any missing data.
There are two ways of handling missing data: dropping the missing values entirely or inputting the missing values. Both methods could lower the accuracy of the data, limit its utility or distort the insights that firms could leverage from the data. Choosing the right approach requires careful consideration and a good understanding of the characteristics of your data and what you hope to achieve with it.
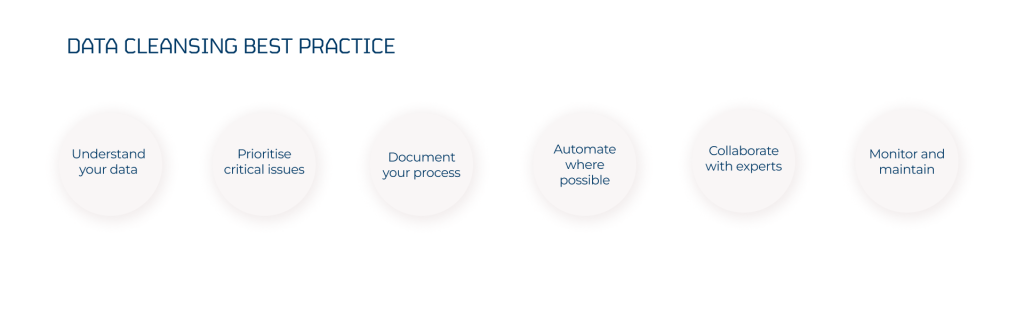
How can Raw Knowledge help?
We are currently deploying an innovative new platform that changes the way data is extracted, processed, and verified.
Our Managed Smart Data platform creates a unified view of disparate data sources and can incrementally improve the structure and quality of the data that flows through it using the power Medallion architecture.
The platform’s Medallion architecture has three layers: bronze, silver and gold. In the bronze layers, raw data can be stored in its original form to preserve data lineage and maintain a clear audit trail. In the silver layer, the data is cleaned, validated and transformed to prepare it for analysis. In the final gold layer, the data is fully processed and ready for a variety of business uses.
By handling data in this manner, our Managed Smart Data Platform helps businesses streamline their operations, make better data-driven decisions, and uncover new revenue streams.